This article is inspired by the shift in the data engineering space over the last few years with the evolution of modern data stack.
Modern data stack is the collection of below best-in-class tools that does wonders together.
- Cloud data warehouse for storing the data (Snowflake, Databricks, Redshift, etc.,)
- Data Ingestion tools for loading data into data lakes (Fivetran, Airbyte, Stitch, etc.,)
- Data modelling tools for data transformation (DBT, Dataform, etc.,)
- Reverse ETL tools to load transformed data back to the application (Make, Hightouch, etc.,)
- BI tools for creating dashboards (Tableau, PowerBI, etc.,)
How Data Engineering played out before modern data stack?
Traditionally, companies have focused on collecting and visualizing data. Data extracts from different source systems are shared through FTP/SFTP to the Unix servers. These files are processed by traditional ETL platforms like Informatica or DataStage and the transformed data is loaded into data warehouse. Data from the warehouse is then extracted, denormalized and uploaded to the BI platforms for visualization.
This entire process of collecting data to visualization has taken up all the energy of the data teams because of the complications and inflexibility in the process. Not only does this remain an expensive process in terms of time and resources, it is also not fast enough to support data driven businesses.
Maintaining the file servers and database servers and keeping up with the upgrades were tedious and data quality issues from the source data were often directly impacting the reports. We did not had much control over data quality other than having some validation checks at the file level using some Unix scripts. Versioning of code was a separate process to deal with as there is no inbuilt structure to support that.
How cloud providers changed this equation?
Cloud providers like AWS, GCP and Azure came up with the idea of providing services that they could manage for you, so you need not worry about maintaining the storage or even maintaining the compute servers. This was a great relief and companies happily adopted the services that cloud providers offered including managing users in the system, access control, storing data in the cloud and the ability to ready data directly from the files in the storage location, ability to use any compute machines without the need to maintain any backend process. Cloud infrastructure later evolved to provide end to end solutions including managing data pipelines, data transfer and storage.
Cloud providers found success in convincing the companies of shared responsibility model for data security. The interesting part is how they charged for the usage on pay as you go model. Price monitoring interface offered with these cloud systems provides greater transparency in pricing. Data handling was better than before, and it reduced the complexity and time and helped companies to focus more on business growth.
How Data ingestion tools made data movement effortless?
Data ingestion tools like Fivetran, Stitch, Airbyte, etc., simplified the data movement between source applications to cloud databases. With basic configuration and a couple of clicks, we can pull data from anywhere and load anywhere. With prebuilt connectors readily available to most of the widely used SaaS applications and databases, data is loaded effortlessly into cloud databases. This also reduced the downtime of data applications and allowed the data team to focus more on the transformation side, creating business value without worrying about data pipeline maintenance. After the evolution of these ingestion tools, companies started moving from traditional ETL to ELT designs.
How cloud databases supported this evolution?
Cloud databases like Snowflake, Databricks, BigQuery have taken it further by supporting integrations from different applications, handling storage and compute better by decoupling them, improving performance across infinite number of concurrent workloads with multi-cluster shared data architecture, providing unlimited scalability, strong governance and security protocols, all that by letting you pay only for what you use.
How analytics tools changed the game of data transformations and added more value?
Analytics made easier with tools like DBT where transformations are created as SQL models, which are then executed to materialize as tables/views in data warehouse. DBT not only completely takes care of “T” part of the ELT and has more features that help data validation to a great extent with source freshness checks and inbuilt data validation checks ensuring data integrity. DBT can handle dependencies seamlessly which is exceptional.
It also supports modular code, version control and continuous integration/continuous deployment (CI/CD). It also helps maintains data documentation and definitions within the system as DBT build and develop lineage graphs. Lineage graphs along with detailed descriptions ensure transparency and enables business users to see through the data flow and the incorporated business logic.
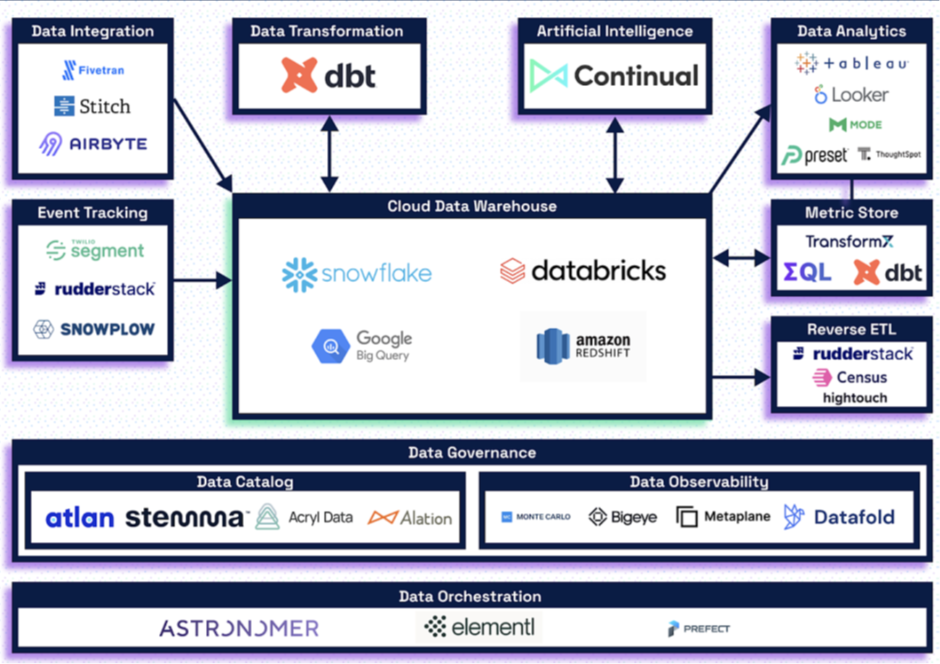
With affordable and easy to adopt tools which include Cloud warehouses (Snowflake, Databricks, BigQuery, Redshift), Data ingestion tools (Fivetran, Stitch, Airbyte), Analytics tools (DBT), and BI tools (Tableau, PowerBI), there’s no reason why any company shouldn’t have a proper data stack setup for informed decision-making processes.
If you are trying to figure out the most applicable data strategy for your business, please write to us at connect@ngenux.com to have some exciting conversations around data related technologies like Data Engineering, Data Science, Machine learning, Artificial Intelligence, Business Intelligence and Visualization.