Are you looking for ways to increase your profits and stay ahead of the competition? One strategy that you might want to consider is using machine learning to optimize your prices.
Price optimization using machine learning involves using algorithms and data analysis to identify the optimal price for a product or service. This helps businesses to maximize their revenue and profits by setting prices that are competitive, but not so low that they sacrifice profitability.
Here is an interesting use case of one of the Ngenux’s customers which is a marketplace for financial service which provides services through freelancers/experts. Without a price recommendation, experts lack visibility into market dynamics and have low confidence on the pricing they quote if it is in-line with client expectations. Likewise, clients have no understanding of the fair market price for services/solutions provided by the experts.
Given that pricing is the leading driver for opportunity conversion and revenue realization, the requirement is to come up with an ML based solution which suggests real – time optimal price for every new opportunity which comes in, maximizing the chances of conversion and driving revenue.
Ideal Solution:
An outcomes-based pricing system enabled by AI algorithms was built. The system consists of 2 Machine Learning models and an optimizer that, when combined, optimizes the win rate and revenue.
Design:
Traditional text books on pricing talk about price elasticity. Price elasticity curves are typically drawn to determine the change in demand for a product or service by changing prices. Unfortunately, this single-minded approach does not guarantee revenue incrementality. One can set the price to zero and generate large demand. However, the revenue will also be zero. Price elasticity to generate demand is important for revenue management, as long as the maximization of demand also maximizes the revenue. The current design maximizes the win rate and also the revenue realized from it. The first ML algorithm predicts the project’s probability of winning at a specified price. The second algorithm then estimates the revenue based on the price. An optimizer is then used to determine the optimal rate, maximizing the win rate and the revenue.
The image below visualizes the system architecture :
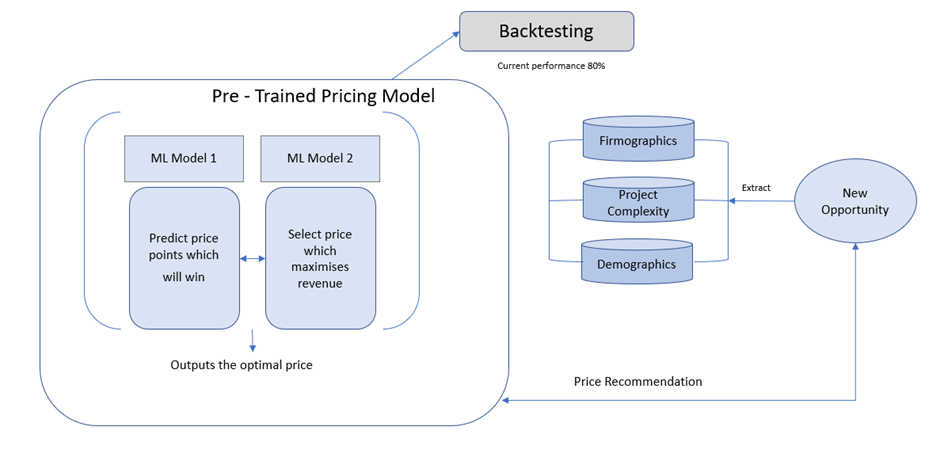
Extraction of price range:
Individual price point not be a desirable output as the expert might want to have a certain flexibility over price range. Hence a price range logic was designed. All the price points, around the optimal price point which predicts a revenue close to the maximum revenue (Around 5% of maximum revenue) is derived as a price range.
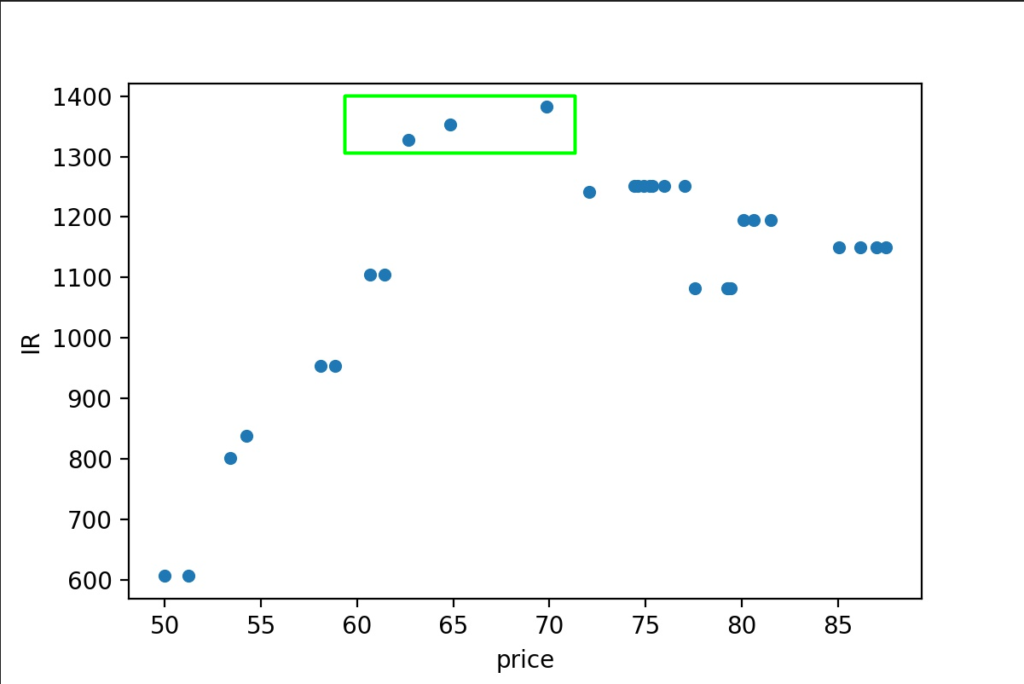
Solution Architecture Diagram
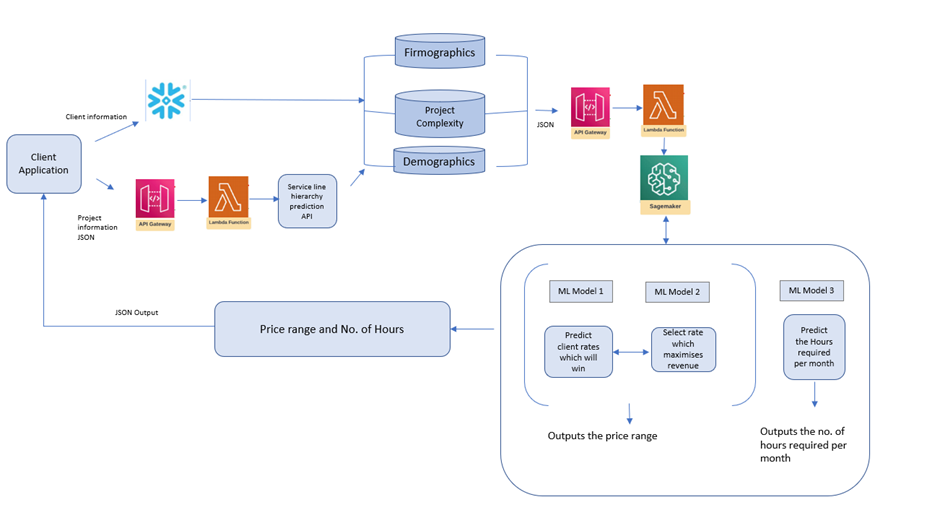
Challenges during implementation:
- Local minima problem:
The optimizer often went through local minima problem. The optimizer would find out a local minima in a given price range and assume it to be the optimal price point. But the optimal price is at the global minima and not at the local minima. This challenge was tackled using the scipy.optimize.basinhopping optimizer instead of using scipy.optimize.minimize which overcomes the local minima problem.
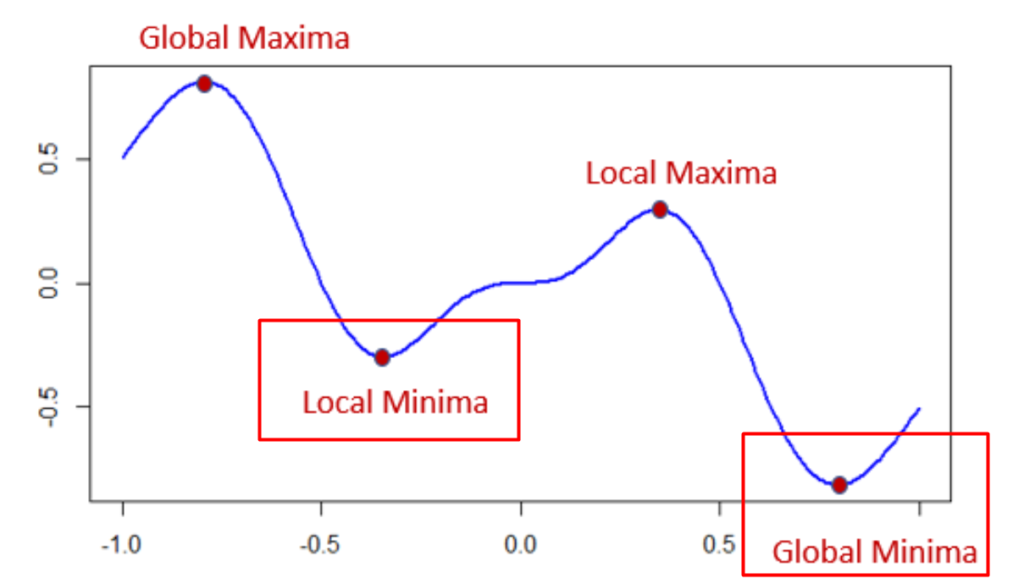
2. API run time:
The business expected API run time was within 5 -7 seconds, but the initial run time for the API was around 35 – 45 seconds. On analysing, it was observed that the major amount of run time was due to the optimizer.This challenge was overcome by adjusting the No. of iterations and the stepsize for the optimizer, such that the error and run time both were at the minimal.

Timeframe for implementing the solution:
The project took an overall of around 6 months to implement. This includes around 2 months for preliminary data analysis , data preparation and feature selection, 3 months for Design, Model building, optimizer building, performance improvement and 1 month to deploy the API.
Metrics/measures used for evaluating the Solution:
The focus of the pricing strategy is to ensure launch and very high revenue realisation in the first 3 months, after which retention and growth are determined largely by expert. Hence, pricing focuses on winning projects that earn the maximum 3-month revenue.
Business outcome / Benefits:
The algorithm could help achieve competitive prices and give the client an edge over other firms. Also, we could see uplifts of around 5% in key business metrics such as profit margins and total revenue. The algorithm also brought coherency to the entire pricing process in the organisation.
Ongoing support / Maintenance of the solution provided:
To capture the changes in the data patterns, retraining of the algorithm is done every 2-3 months. A pricing performance dashboard is built to track the efficiency of the algorithm.
In summary, if you want to increase your profits and stay ahead of the competition, consider using machine learning to optimize your prices. By analyzing data and using algorithms, you can set prices that are fair, competitive, and profitable. You can get in touch with us at connect@ngenux.com to know more about our business solutions to supercharge your business decisions and boost revenue.
Cover image courtesy: www.freepik.com