In today’s fast-paced digital landscape, businesses and organizations need to process and analyze vast amounts of data in near-real-time to stay competitive. This is where near real-time data streaming comes in.
Near real-time data streaming involves the continuous processing of data as it’s generated, rather than waiting for the data to be stored in a database before analysis. This allows for faster and more accurate insights, which can be critical for businesses in industries such as finance, healthcare, and e-commerce.
One of the key advantages of near real-time data streaming is the ability to respond quickly to changing conditions. For example, financial institutions can use real-time data to detect fraud, and e-commerce companies can use it to provide personalized recommendations to customers. By processing data as it’s generated, businesses can identify trends and patterns more quickly, enabling them to make better decisions and stay ahead of the competition.
But implementing a near real-time data streaming system isn’t always easy. It requires the right infrastructure, including high-speed networks, distributed computing systems, and data storage solutions. It also requires expertise in data management and processing, as well as the ability to manage and analyze large volumes of data in real-time.
In this blog, we’ll explore a Near Real-Time Data Streaming use case successfully implemented at one of Ngenux’s customers in the Satellite Radio space. Let’s look at the key benefits achieved, challenges faced, best practices implemented and the business fruition derived. We’ll also look at the leading technologies and platforms utilized in managing the real-time data streaming.
Business Overview
A Satellite Radio Company has started an initiative called LDAX (Listeners Data Exchange) to collect, store and to progressively integrate all their listeners data to create a Single Authoritative View across the enterprise. It will stitch customer identities across the systems with a unified ID and provide up-to-date snapshots of their attributes, behaviors, interactions, transactions and listening usage across any device.
LDAX DaaS (Data as a Service) is the API component of the LDAX eco-system serving high concurrent low latency APIs. It also offers both in-memory and persisted datastores for low SLA API requests.
Business Problem Statement
The current On-Premises system is storing different events that are coming from different source systems, has a very low performance to process 200 million records. Due to sub optimal performance, downstream applications like customer support systems do not have up to date information about their customers which is resulting in poor customer experience.
Solution Proposed
Proposed solution uses Amazon Kinesis Streams to support applications that process and analyze streaming data for specialized needs. In Kinesis Streams, we first create a stream and specify the number of shards that are needed (A shard is a unit of capacity in a Kinesis stream, and you specify the number of shards when you create a stream). Once a stream is created, we can put data records into the stream by using the Kinesis Streams API, and the data in the stream is available to be processed within a few seconds. This has helped the system to scale and handle large number of input requests with out any latency issues
Solution Architecture Diagram
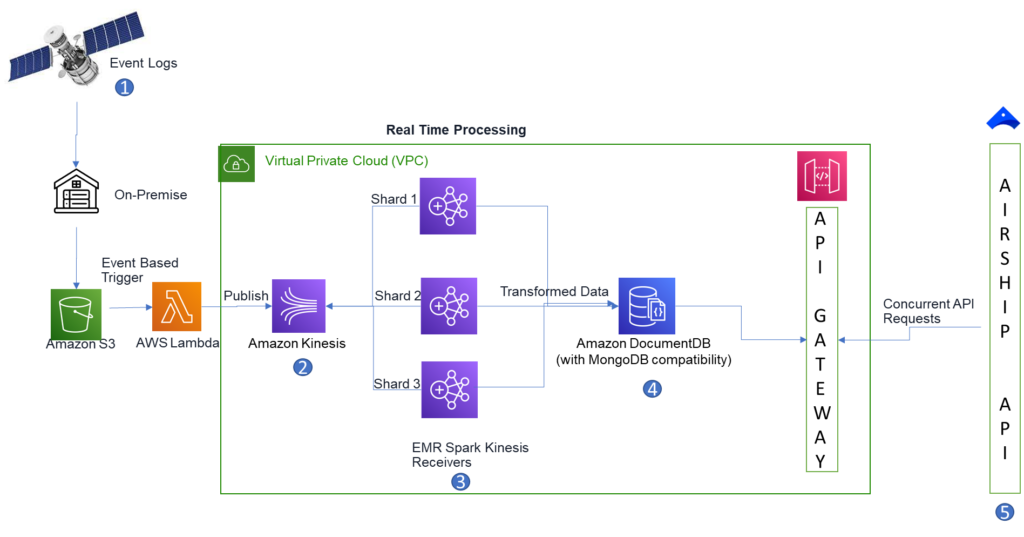
1. Event Logs containing Users Usage Information which are getting generated continuously from the Users devices is captured and loaded to a storage location in AWS s3.
2. Amazon Kinesis Streams will act as Events Collector to which these events get published from a Lambda function.
3. Apache Spark Streaming component running in an EMR Cluster does the Data Processing by consuming from Kinesis Streams acts as Subscriber.
4. Once the Data is Processed, it will be written to Amazon DocumentDB which is a NoSQL database acts as a Sink.
5. Airship, a marketing application is the end user that provides variety of features allowing the business to send targeted and relevant messages to their customers through various channels based on these real time data that is accessible over APIs.
Technology/Frameworks used for Implementing the solution
- Amazon Kinensis
- Spring Boot
- AWS Lambda
- API Gateway
- Document DB
Challenges faced during implementation and resolution
- API Gateway couldn’t handle volume of concurrent requests from Airship application.
- Resolution:
- Increased Rates Per Second Throttling limit in API Gateway
- Increase in response time due to Lambda Cold Start
- Configured Provisional Concurrency to Lambda such that few instances are always active to handle incoming requests.
Business outcome / Benefits
Our solution has resulted in availability of up-to-date information about customers in their customer support application which has resulted in higher customer experience and hence improved CSAT Scores.
Adoption of the solution
This real time solution is backbone of the search APIs used by call center and customer support teams of the organization now catering to more than 200 million requests
This solution became a Standard across different teams within the Organization
Ongoing Support after production release
Ngenux has setup a Monitoring system to continuously monitor the key performance metrics, such as data throughput and processing latencies, to ensure that the solution meets business requirements.
In conclusion, Near Realtime Data Streaming is a crucial aspect of modern data processing, and with our cutting-edge technology and dedicated support team, we can help businesses of all sizes and industries achieve their data goals faster and more efficiently. If you’re looking for a reliable and efficient Near Realtime Data Streaming solution, please reach out to us at connect@ngenux.com to have some exciting discussions around Data Engineering and Data Analytics.