In today’s data-driven world, organizations are constantly looking for ways to improve their data management processes. The traditional approach of centralizing data in a data lake or data warehouse has proven to be inadequate for large-scale data sharing and collaboration. To address these challenges, a new paradigm has emerged: Data Mesh.
What is Data Mesh?
Data Mesh is a relatively new architectural paradigm for organizing and managing data in large, complex organizations. The basic idea behind Data Mesh is to treat data as a product, rather than a byproduct of software development. This means that data is owned and managed by the teams that create it, rather than being centralized in a single data team. Each team is responsible for the quality, availability, and usability of the data they produce, and they are incentivized to collaborate and share their data with other teams.
To enable this kind of decentralized data ownership and management, Data Mesh relies on a set of architectural principles and patterns, including domain-driven design, bounded contexts, APIs, and self-service data platforms. These patterns allow each team to define their own data domains, create APIs to expose their data to other teams, and build their own data products using data from other teams.
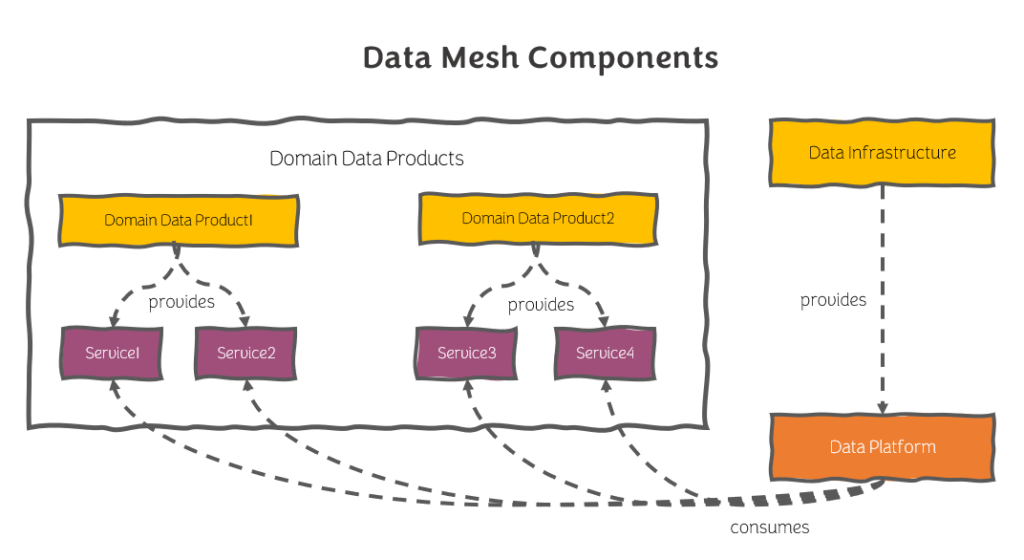
The benefits of Data Mesh include faster time-to-market for data products, better data quality and governance, increased collaboration and alignment between teams, and improved agility and flexibility in responding to changing business needs.
While Data Mesh is still a relatively new concept, it has already gained traction in the industry, with several large organizations adopting it as their preferred data architecture.
Challenges with Traditional Approach
Data Engineering has evolved significantly over the years, with new technologies and techniques emerging to meet the evolving demands of data management and processing. Below are some of the challenges faced with Traditional Approach:
- Centralized Data Storage: Data is stored in a single database or data warehouse, making it difficult to manage large volumes of data. Additionally, having a single point of failure can increase the risk of data loss or system failure.
- Data Overload: Monolithic architecture also suffers from data overload, where the system is inundated with data from various sources in multiple formats, making it difficult to process and analyze data effectively. The system may struggle to handle the sheer volume of data, leading to poor data quality and accuracy.
- Data Silos: Upon examining the work of those who are involved in building and managing data platforms, it becomes clear that the team members are specialized data engineers who are largely disconnected from the operational aspects of the company that generate or use data to make decisions. These data platform engineers are typically divided into separate teams based on their technical expertise with big data tools, and the organizational structure often lacks integration with the company’s business operations and domain knowledge.
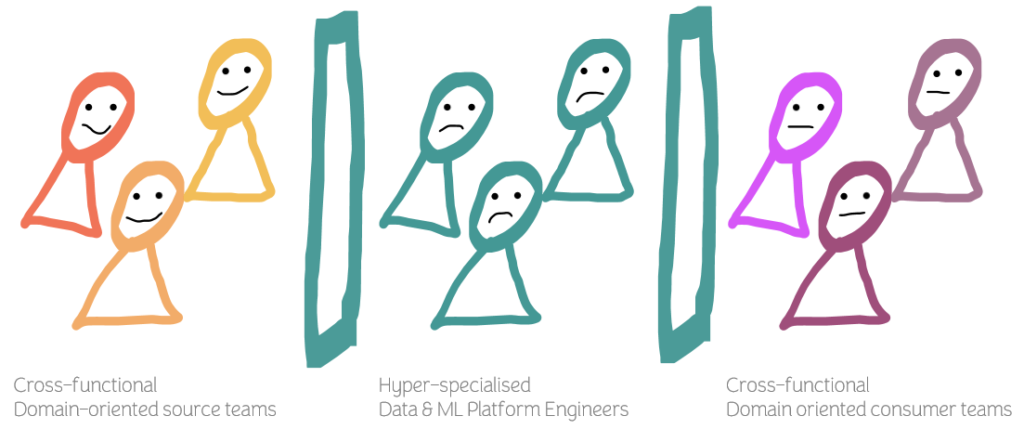
Key Components of Data Mesh
Data Mesh consists of several key components that work together to create a decentralized data architecture. These include:
- Domain-Driven Design: A design approach that aligns technical and business domains, enabling the creation of a self-serve data infrastructure.
- Platform Thinking: An approach to software development that focuses on creating reusable and scalable infrastructure to support multiple domains.
- Data Products: A concept that treats data as a product, with defined interfaces, and ownership, ensuring data quality and consistency.
Advantages of Data Mesh
There are several benefits of implementing Data Mesh in a data engineering space. These include:
- Faster time to market: By enabling teams to work independently and with greater autonomy.
- Increased Data Ownership: By aligning business domains with technical domains, data ownership is clearly defined, reducing the risk of data silos and improving data quality.
- Improved Data Accessibility: By treating data as a product, Data Mesh enables self-service data access, reducing the dependence on centralized data teams.
- Enhanced Data Collaboration: By creating a decentralized data architecture, Data Mesh enables cross-functional teams to collaborate more effectively on data initiatives.
- Scalability and Flexibility: Data Mesh’s decentralized architecture enables organizations to scale their data management processes as their data grows, and accommodate changes in their data landscape.
Implementing Data Mesh in Your Organization
Implementing Data Mesh in an organization requires a significant change in mindset and approach to data management. Here are some key steps to consider when implementing Data Mesh:
- Define data domains: The first step in implementing Data Mesh is to define the data domains within your organization. This involves identifying the different areas of your business that generate, use, or need access to data.
- Establish data governance: To ensure that your Data Mesh implementation is successful, it is important to establish data governance. This involves defining policies, procedures, and processes for managing and controlling access to data.
- Create self-serve data infrastructure: Data Mesh is based on a self-serve model, where data consumers have direct access to the data they need. To support this model, it is important to create the necessary infrastructure to enable self-service data access.
- Implement data products: In Data Mesh, data is treated as a product. This involves defining the data products that need to be created, as well as the processes for creating, maintaining, and delivering these products.
- Develop data integrations: In a Data Mesh architecture, data is often integrated from multiple sources. It is important to develop the necessary integrations to ensure that data is accessible and usable.
- Monitor and manage data quality: In Data Mesh, data quality is critical to success. To ensure high-quality data, it is important to establish processes for monitoring and managing data quality, as well as addressing any issues that arise.
- Foster a culture of data-driven decision-making: Finally, to ensure that Data Mesh is successful, it is important to foster a culture of data-driven decision-making. This involves educating stakeholders on the importance of data, as well as encouraging the use of data in decision-making processes.
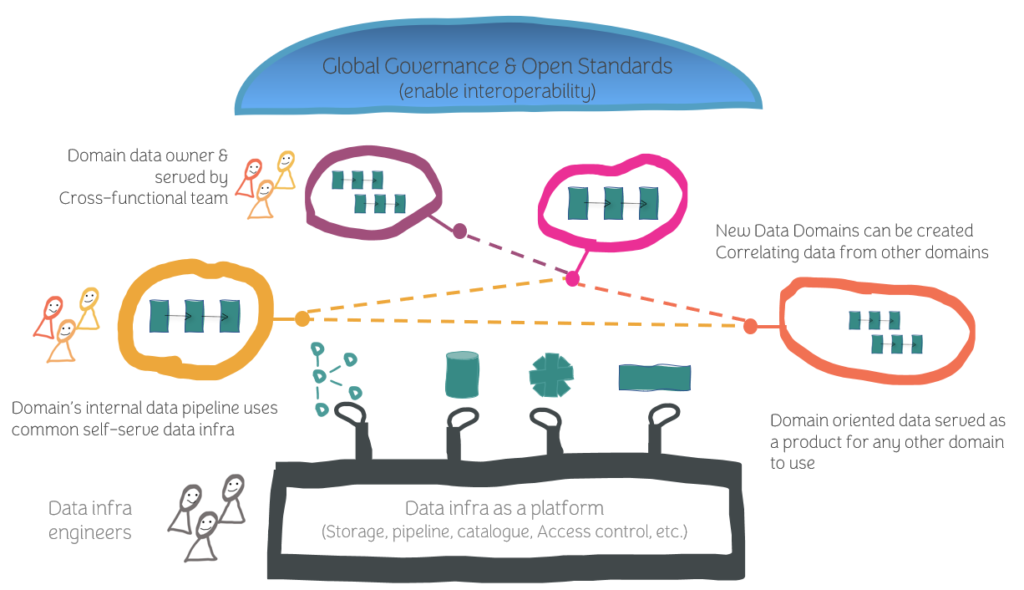
The data mesh platform is a purposefully planned distributed data architecture that is supported by a shared, standardized self-serve data infrastructure and is governed centrally for interoperability. Building a Data Mesh can be challenging, as it requires a significant shift in organizational culture, as well as the adoption of new architectural patterns and practices.
As a leading provider of cloud-native technologies and services, Ngenux can provide the expertise and recommend tools to organizations to build and manage their Data Mesh. Partnering with Ngenux for implementation for service mesh and data platforms, organizations can accelerate their adoption of Data Mesh and realize its many benefits.
Ngenux’s approach to building Data Mesh involves leveraging service mesh technology to provide a scalable and flexible platform for teams to build and manage their data products. Ngenux also offers a range of data platform services, including data lakes, data warehouses, and data pipelines, that can be integrated into the service mesh.
To know more about Ngenux and its offerings, please get in touch with us at connect@ngenux.com.