With the explosive growth of machine learning and AI, we are seeing an increasing number of companies trying to improve their business by adopting these practices. As per a report from MIT, digitally mature firms are 26% more profitable than their peers. According to Accenture, data-driven companies grow at an average of more than 30% annually. Also, more than 60% of the 2,000 largest consumer goods enterprises indicate they are counting on artificial intelligence to manage their supply chain initiatives by 2024, expecting an increase in productivity of more than 20%. Despite integrating all the latest data-driven technologies, the success rate of these projects is very poor. According to Gartner, only 20% of analytic solutions deliver business outcomes. Most of the machine learning projects never make it to production. There can be multiple causes for the failure of these projects, out of which the one we’ll be discussing here is known as Model Drift.
The world around us is changing at an unprecedented pace. To keep up with the trend you need to constantly update yourselves with the advances that are happening with time. You need to rethink your business strategies and plan if you want to gain an edge over the competitors. Things that might have seemed relevant in the past might have become obsolete now. A machine learning model which was built some time ago might have degraded over time, often known as model decay. Before diving in deep, let’s start by understanding the meaning of Model drift and then in the later sections you will also see how this drift impacts business over time and how we should deal with it.
What is Model drift?
A model is said to be drifted when it loses its predictive power due to alterations in the environment. Importantly, whenever a model drift occurs it can impact an organization negatively over time or in some cases its immediate . According to 2021 IBM Global AI Adoption Index 2021, 62% of businesses surveyed cited ‘’Unexpected performance variations or model drift’’ as the biggest AI model performance and management issue businesses are dealing with. An ML system trained up and ready to go may give great results when initially deployed, but its performance can degrade over time due to drift. The drift can be caused due to changes in the data with time or changes in the relationship between variables.
Understanding Concept drift and Data drift
Broadly speaking the drift can be classified into two categories – Concept drift and Data drift. Concept drift is where the relationship learned by our model between the independent and dependent variables has changed over time. In other words, the target we want to predict has changed over time. The same input x now demands a different prediction y. For example, if we had a regression model which predicts the price of a house based on area and number of bedrooms. The model accuracies could have come down due to inflation which in turn caused the prices to rise.
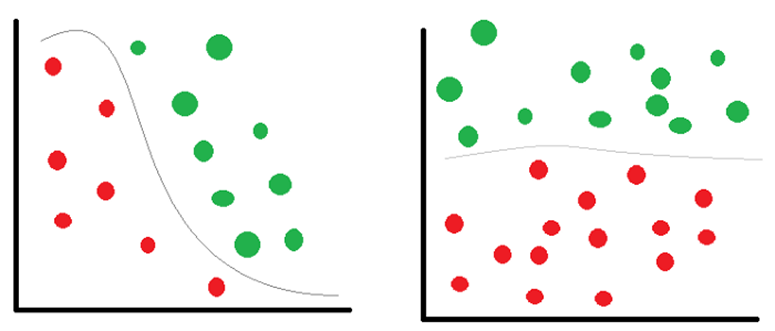
Concept drift is largely caused due to seasonal variations over time. Let’s look at another example to understand better. We know that personalization is the key to success for most of customer-centric businesses. Let’s say you build a recommendation system that provides personalized search results or recommends relevant products based on the user’s shopping pattern. You notice that the user shopping behavior has changed over time for some reason, it could be due to a life event like marriage, pregnancy, relocation to a different city or it could be due to a pandemic like COVID-19. These kinds of events drastically change the user’s shopping habits, so a personalization system built today might not be so relevant after a few years.
Data drift occurs if the underlying distributions of the features have changed over time. This can happen due to reasons such as seasonal behavior or change in the underlying population. For example, if you had a model that forecasts the demand for electricity from historical data. The accuracy of predictions now might have changed due to climate change, which is causing unprecedented changes to the weather nowadays.
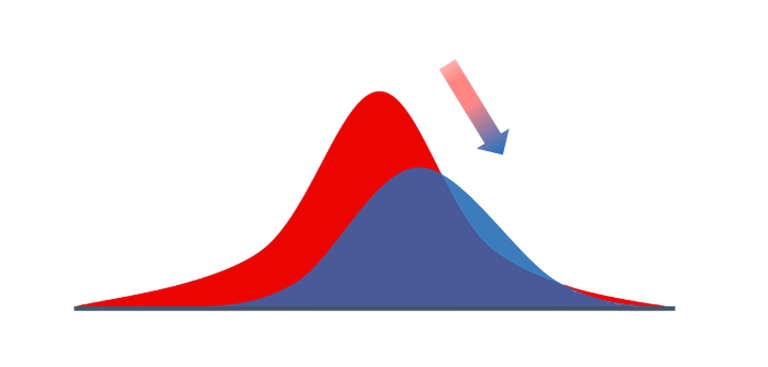
To have a better understanding of data drift let’s look at some more cases. If something changes upstream, then you can have a data drift. For example, an IoT sensor used for collecting data has been replaced due to which the unit of measurement has changed, or we have a malfunctioning sensor constantly reading a value of zero. Sometimes the drift can be natural, the distribution and statistical properties of data might have changed due to varying seasons. If the relationships between the features change over a period, then also we can expect our data to drift. Therefore, it becomes an important task for us to monitor both our data and model frequently after deployment.
Pace at which drifts impact business
Drifts which we have discussed above can be sudden, gradual, or perhaps recurrent in nature. In cases like the COVID-19 pandemic, it was a sudden and abrupt change affected several sectors like healthcare, eCommerce, finance, small-scale enterprises, and many more. Such a type of change is usually due to external driven factors and are generally unpredictable and unavoidable.
Just 2 years after the official start of the pandemic, we are noticing a rise in inflation across the globe which might also impact our model used for predicting prices. This kind of change can be considered as slow and gradual process, and we can see its impact on results over course of time.
Ecommerce sales like Freedom Sale, Big budget sales, and others that happen during a specific period of a year, are often periodic in nature. So a different model specifically trained on the Freedom Day sale data is used during the time of that event.
How to detect a drift?
The most accurate way to detect model drift is by comparing the predicted values to the actual values. The accuracy of a model worsens as the predicted values deviate farther and farther from the actual values. A common way to measure accuracy is F1-Score which elegantly combines two otherwise competing metrics known as precision and recall.
The Kolmogorov-Smirnov (K-S) test is a nonparametric test and can be used to compare cumulative distributions of two data sets.
Population stability Index (PSI) is a metric used to measure how a variable’s distribution has changed over time. There are other statistical distance methods like Kullback-Leiber Divergence, Jenson-Shannon Divergence, and many others which can be used to detect the drift. I have only a discussed a few here.
What do you do after detecting a drift?
One way to address drift is to periodically re-train the model at different instances of time if there is any model degradation below a threshold.
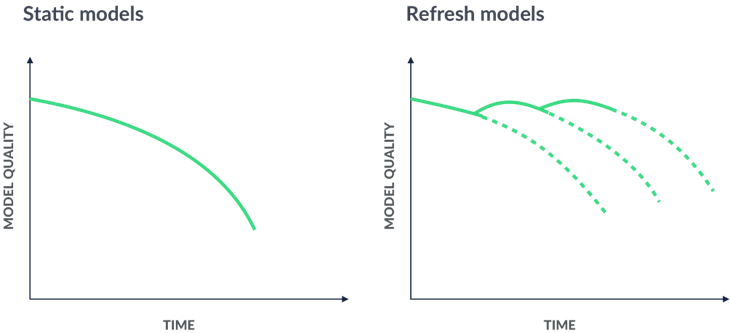
For real-life applications run on streaming data, Online learning is the most prominent way to prevent concept drift as your model learns on the fly. These are the two most effective methods for tackling drifts in the model. There are some other methods like dropping the feature or ensemble learning with weighted models which might also help.
Finally, would like to conclude by reminding that business dynamics change from time to time, and you should constantly review the relevance of the existing data and models that you set up in production to avoid any performance issues that may occur in future. To find out how Ngenux can help you keep ahead of machine learning drift, contact us at connect@ngenux.com and have an educated discussion with our experts.