Introduction
In today’s fast-paced world, data-driven decision-making (DDDM) reigns supreme. However, there are obstacles that hinder even the most talented data scientists, engineers, and analysts from providing effective and efficient data-driven solutions. Weeks are wasted waiting for required data, Infrastructure management becomes a challenge, and tracking changes becomes a nightmare. Enter DataOps, the game-changing solution that transforms data management into a streamlined and collaborative process. With DataOps, teams can unlock the true potential of their data, making informed decisions faster and more effectively. So, say goodbye to bottlenecks and hello to a new era of seamless data-driven success. Get ready to embark on your DataOps journey today!
According to Gartner’s latest research on Data Management, DataOps is gaining momentum as an approach to increase the speed, efficiency, and quality of data-driven decision-making.
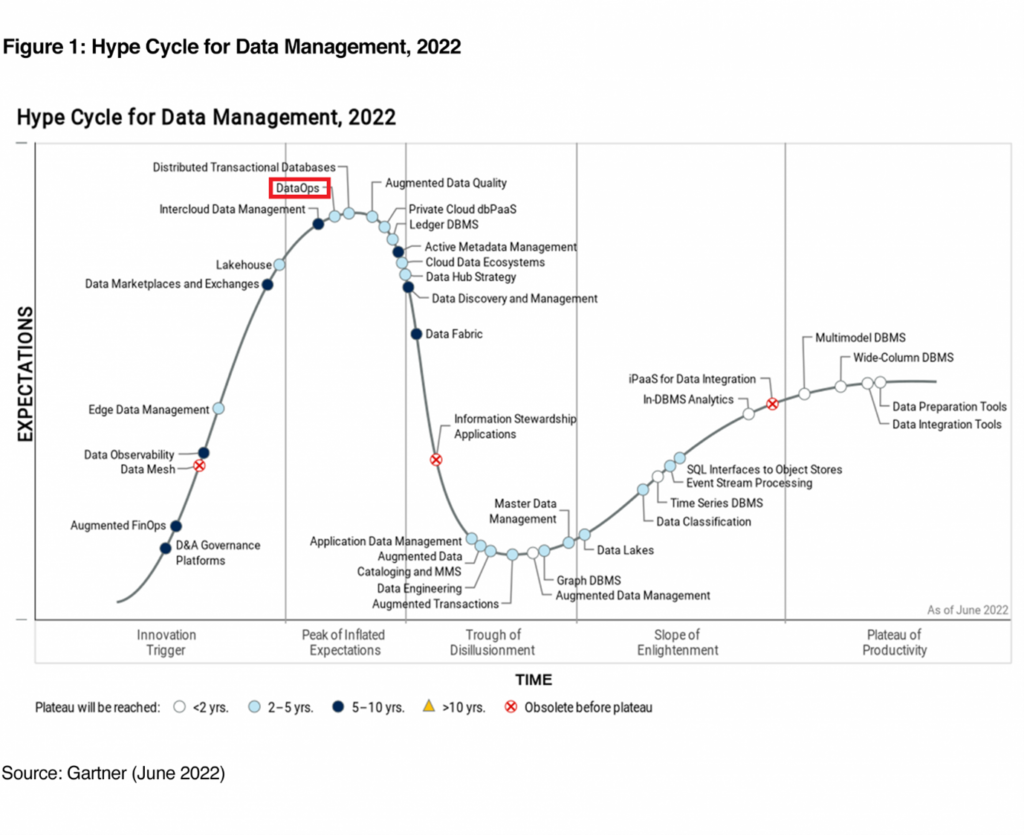
What is DataOps
DataOps can be defined as a set of practices and tools that help organizations manage data more efficiently, automate data operations, and improve the quality of their data. It is a methodology that assembles data engineers, data analysts, and other stakeholders to work together on projects using data. Some examples of DataOps in action are presented below which may be relatable to certain real-time scenarios.
Use Cases in multiple domains
- A Retail company that uses data from its online store to optimize its pricing strategy. The company collects data on customer purchases, website traffic, and product reviews, and then uses DataOps tools to transform and analyse this data in real-time. With DataOps, the company can quickly identify trends in customer behaviour and adjust its prices, accordingly, leading to increased sales and revenue.
- A Healthcare organization that uses DataOps to improve patient outcomes. The organization obtains data from a variety of sources, such as electronic health records, medical imaging systems, and patient monitoring devices. With DataOps, the organization can automate the process of collecting and transforming this data, enabling faster diagnoses, better patient outcomes and more individualised treatment protocols.
- A Financial institution that uses DataOps to comply with regulatory requirements. The institution collects data on customer transactions, trading activity, and regulatory compliance systems. With DataOps, the institution can automate the process of managing this data, ensuring its accuracy and consistency, and providing regulators with the data they need to ensure compliance.
In all of these examples, DataOps helps organizations manage their data more efficiently, their ability to make decisions improves, giving them a competitive advantage. By streamlining the data pipeline, automating data operations, and improving data quality, organizations may maximise the potential of their data to improve business results.
Key principles of DataOps:
1. Collaboration: DataOps emphasizes the importance of collaboration between different teams involved in data management, including data engineers, data scientists, and business analysts.
2. Automation: Automation is a key component of DataOps, as it helps to reduce the risk of errors and improve efficiency. By automating repetitive tasks such as data ingestion, cleaning, and transformation, DataOps teams can focus on more complex and valuable activities.
3. Continuous Integration and Delivery: DataOps seeks to ensure that data processing and analysis is done in a continuous and iterative manner, with regular updates and improvements made to data pipelines.
4. Agile Methodology: DataOps adopts an agile methodology, which emphasizes flexibility, adaptability, and responsiveness to changing requirements and business needs.
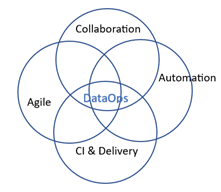
Challenges of Traditional approach:
Increasing data volumes: As organizations collect more and more data, managing and processing that data can become increasingly complex and time-consuming. DataOps helps to streamline data processing by automating and standardizing data management tasks.
Data silos: In many organizations, data is stored in different systems or databases, which can create data silos that make it difficult to access and analyze all of the data in a single place. DataOps helps to break down these silos by integrating data from different sources and creating a centralized data repository.
Inconsistent data quality: Inaccurate or incomplete data can lead to incorrect insights and decisions. DataOps helps to ensure data quality by implementing automated data validation and quality checks.
Slow data delivery: Traditional data management processes can be slow and time-consuming, which can slow down decision-making processes. By automating several data processing and analysis procedures, DataOps speeds up data delivery.
Lack of collaboration: In many organizations, different teams and departments work in silos, which can make it difficult to collaborate effectively on data-related projects. DataOps helps to promote collaboration by creating cross-functional teams that work together to manage and analyse data.
Advantages of DataOps:
1. Improved Efficiency: By automating and streamlining data-related processes, DataOps can help organizations save time and reduce costs.
2. Better Collaboration: DataOps encourages collaboration between different teams involved in data management, which can lead to better insights and decision-making.
3. Faster Time-to-Insights: By adopting a continuous integration and delivery approach, DataOps can help organizations get insights from data faster, enabling them to make more informed decisions.
4. Reduced Risk of Errors and Data Loss: By automating and standardizing data-related processes, DataOps can help organizations reduce the risk of errors and data loss.
Implementing DataOps in your Organization
Implementing DataOps requires a combination of technical skills, organizational change, and cultural transformation. Here are some steps you can take to implement DataOps in your organization:
1. Define your DataOps Strategy: Start by defining your DataOps strategy and objectives. This should involve identifying the key data-related processes that need improvement, as well as the teams and stakeholders involved.
2. Establish a DataOps Team: Create a dedicated DataOps team that can oversee the implementation and execution of your DataOps strategy.
3. Automate Data Processes: Identify the key data-related processes that can be automated, such as data ingestion, cleaning, and transformation. Use tools such as Apache Airflow, AWS Glue, or Azure Data Factory to automate these processes.
4. Integrate Data Silos: Integrate data silos and create a unified view of data using tools such as Apache Kafka or Apache NiFi.
5. Adopt a Continuous Integration and Delivery Approach: Adopt a continuous integration and delivery approach for your data pipelines, using tools such as Jenkins or GitLab.
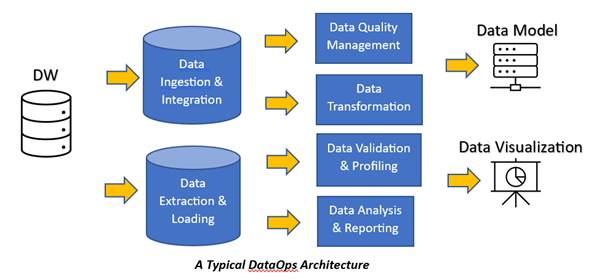
Conclusion:
Overall, implementing DataOps requires a significant investment in both technology and organizational culture. However, the benefits of improved data quality, efficiency, and agility can be substantial, and can help organizations to achieve their data-driven goals more effectively.
The Team at Ngenux can work together identify the specific goals for implementing DataOps. It can define the processes and workflows required. Our team can help evaluate a data integration platform, data quality tools, analytics, and BI Tools. And lastly, work on monitoring and measuring its effectiveness. To find out how Ngenux can help you with your DataOps journey, contact us at connect@ngenux.com and have an educated discussion with our experts.