Freelancers made up 37% of the US workforce in 2021 and the percentage is still growing. Number of platforms offering freelancers for variety of services are growing exponentially across the Globe. These platforms has to provide best matching freelancers to their client’s requirement to run their business successfully. Below is the case study on how we have leveraged NLP techniques to solve match making problem for one of our client.
WHO is the CLIENT
Our client is one the leading digital marketing talent provider, where they match companies with marketing experts
Business understanding / Business problem
Our client was experiencing exponential growth in their business, and they are receiving large number deals on their platform, but their process of matching an expert to client’s requirement is manual and not a scalable solution to meet the incoming flow of deals , because of which they are loosing the business.
Existing client process, sales team documents deal requirements and talent operations team finds right freelancer mapping all the match attributes, though this process was automated to the extent possible, but client is challenged to find freelancers with true availability and willingness to take up new assignment which was time consuming to close one match and the major concern was matchmaking performed by multiple talent operations personnel which makes this process inconsistent, and very subjective to implicit biases.
Solution PROVIDED
Data received in summary listed below. We automated the matchmaking based on fit to client requirements using an AI based keyword match algorithm. Using a natural language understanding algorithm trained on client’s business. This algorithm read the client’s requirements, the notes written by the salesperson during discovery call, extract the entities from both freelancers and deals data, classifying them into appropriate entity categories, and match it to the right freelancer by reading the profiles for all the eligible freelancers. The end goal is to extract the entities from both freelancers and deals data and classifying them into appropriate entity categories prior scoring and ranking the profile for each deal requirement.
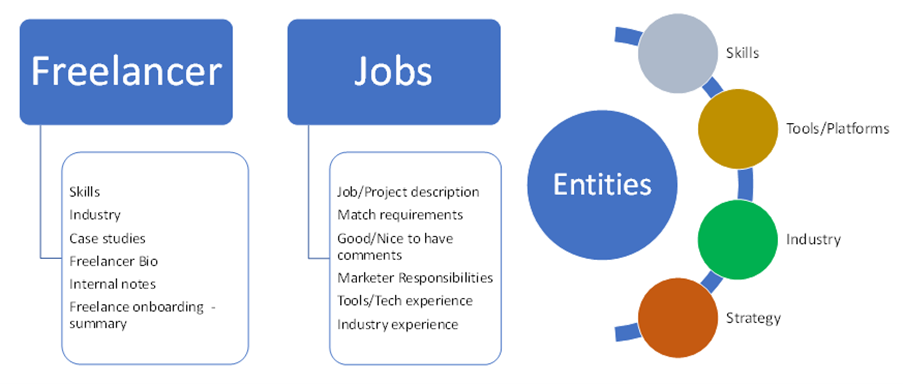
Named Entity Recognition:
NER essentially extracts entities into a pre-determined category. The entity can be generic like organization, person, location, time, etc., or a custom entity depending on the use case like healthcare terms, Programming languages, product category, etc.
In most cases, the NER model is a two-step process:
1) Detection/Recognition of Entities: This step involves detecting a word or string of words that form an entity. For example, “Andaman and Nicobar Islands” it is a single entity made up of four words or tokens.
2) Categorization – In this step, category need to be created first, such as name, location, event, organization, skills, tools etc. Any kind of categories can be created that caters to your needs. You can also provide granular rules for which entities belong to which category in instances of ambiguity or task-specific ontologies and taxonomies.
Here is an example snapshot of how a NER algorithm can highlight and extract entities from a given text:
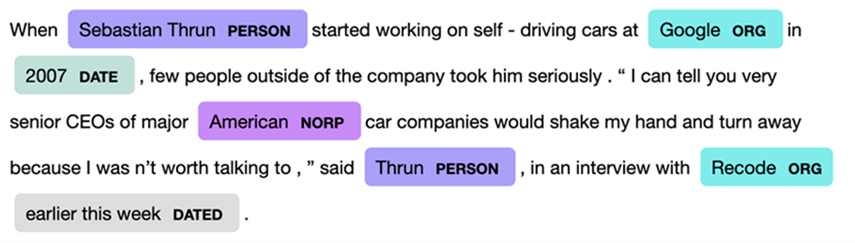
Methods of NER:
1. Dictionary-based:
This is the simplest NER method. In this approach, a dictionary containing vocabulary is used. Basic string-matching algorithms check whether the entity is present in the given text against the items in the vocabulary. This method is generally not employed because the dictionary that is used is required to be updated and maintained consistently.
2. Rule-based:
In this method, a predefined set of rules for information extraction is being used which are pattern-based and context-based. Pattern-based rule uses the morphological pattern of the words while context-based uses the context of the word in the given text.
3. Machine learning-based:
This method solves a lot of limitations of the above two methods. It is a statistical-based model that tries to make a feature-based representation of the observed data. It can recognize an existing entity name even with considerable spelling variations.
The machine learning-based approach involves two phases for doing NER. The first phase trains the ML model on annotated documents. In the next phase, the trained model is used to annotate the raw documents. The process is like a normal ML model pipeline.
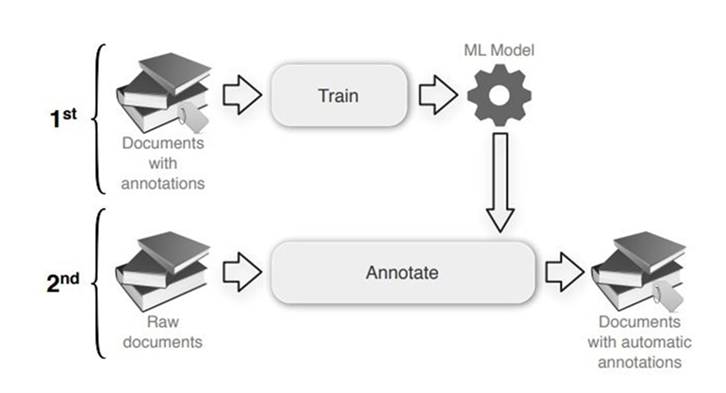
ML Models can be build using one of the 3 approaches available
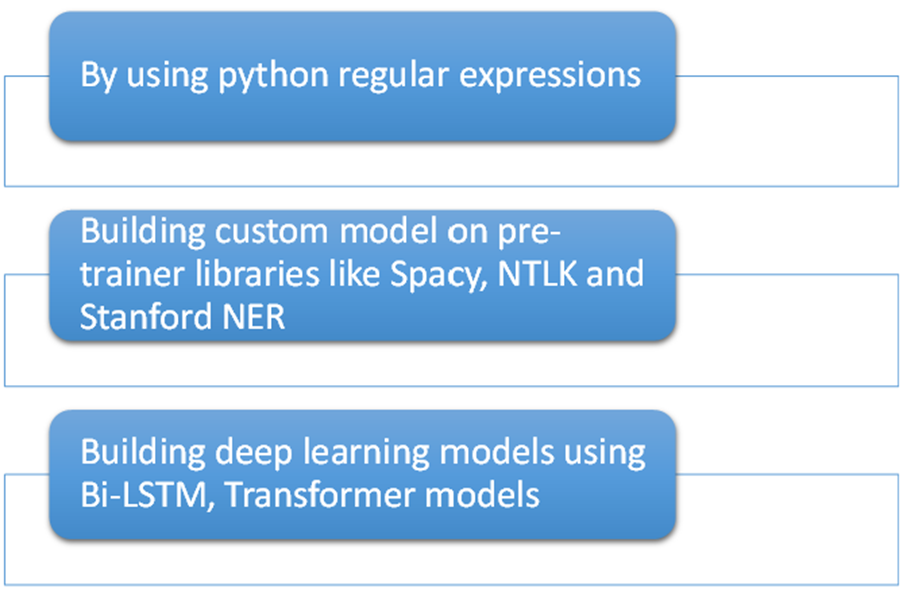
- Custom NER model:
Spacy is an open-source Natural Language Processing library that can be used for various NLP tasks. It provides pre-trained NER model to extract generic entities such as person names, organizations, locations etc. This NER model will not be able to extract domain specific entities for marketplaces like tools, skills, strategy, and industry entities.
Building an accurate in-house custom entity recognizer can be a complex process and requires preparing large sets of manually annotated training documents and selecting the right algorithms and parameters for model training.
We created custom NER model, and it follows below steps:
Define Entity categories:
Used KeyBERT and few NLP techniques for identifying relevant keywords and classifying them into appropriate category as per the marketplaces industry.
Creating Annotated Data:
Once we defined entities and categories, we use them to label data and create training dataset. This is called data annotation.
Spacy requires training dataset in below format
(Sentence, {entities: [(start, end, entity_category)]})
For ex:
(‘Marketer should have ecomm SEO experience.’,{‘entities’: [(21, 26, ‘Industry’), (27, 30, ‘Skills’)]})
There are many data annotation tools available in the market such as Doccano, Prodigy, etc. But they do have free service limitations in terms of number records processing.
Another very important thing we need consider is data privacy issue, here we ought to share the data for annotation, eventually leading to violating GDPR rules.
The Ngenux AI team has spent decent time on R&D for novel techniques such as weak supervision and programmatic labeling to automate training data creation and developed own script to do data annotation and creating training dataset, which ultimately saved costs to business and made sure data privacy followed.
Model Training with flexible options:
We used latest spacy CLI formats and trained on various spacy models. Coming to the system performances we had used high-end (we can even give the configuration) deep learning machines for training the models for several epochs. Extracted keywords from deal/job requirement and freelancers using Google Colab pro (we can even give the configuration).
The matchmaking algorithm creates a list of freelancers who are the best functional fit for a client. The algorithm ranks the freelancers based on their functional fit in descending order. The universe of freelancers selected for matchmaking is the pool of available freelancers at the time of matchmaking.
A good matchmaking algorithm would recommend the best matches in the top ranks. Furthermore, lagging indicators of a good functional fit are “conversion rates”, and “revenue from the deal for which the freelancer is matched.
A well-matched freelancer will be accepted by the clients readily. In other words, the top matches will be accepted by the clients, thereby obviating the need for client to cycle through multiple freelancers before finding the one that fits the best. For example, if client had to reach out to 10 freelancers before one was accepted by the client, then the conversion rate would be 10%. If the matchmaking algorithm can surface that freelancer in the top 5 recommendations, and client had to reach out to only those 5, before the client accepted one of them, then the conversion rate would be 20%.
A freelancer who is a good fit for the job would also tend to generate high revenues. While this is not guaranteed because several other factors, out of matchmaking’s control, come into play for revenue generation, this would be a desirable outcome.
Therefore, our test of the algorithm looks for the following 3 things:
- Did the freelancer who was selected by the client in prior months, through manual matchmaking, recommended by the algorithm in the top ranks?
- If we divide the recommendations made by the algorithm into cohorts of ranks (1-5, 6-10, 11-20 etc.), then do the freelancers in the top cohort have a higher conversion than the freelancers in the lower cohorts?
- Do freelancers in higher cohorts have a higher weekly revenue as compared to freelancers in the lower cohorts.
Results and Deployment
- Comparison with historical matches for accuracy calculations
- Conversion rates
- Avg. Revenues and showed trade of prize using AI
The matching algorithm will output a list of freelancers along with their ranks derived from weighted scores.
The deal will be passed from the matchmaking orchestration layer to the algo APIs. The deals data will be picked from the feature store and attributes will be extracted in real time. Logs and outputs would be stored back in the feature store for computing model performance.
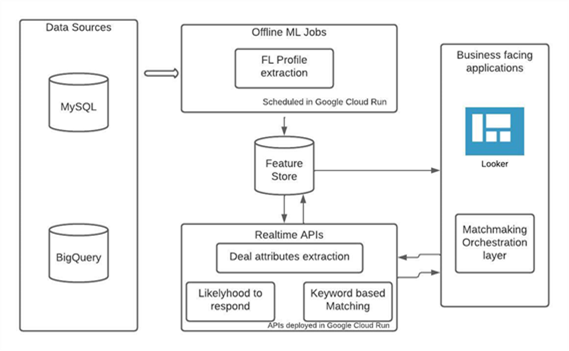
Business outcome
Implemented an AI based matchmaking algorithm, the business goal optimized by this is:
– Fit to clients’ requirements
– Time to match within 24 hours
– Ability of the freelancer to maximize revenue
– Provide equal opportunity for all Freelancer
The algo helped client to improve the turnaround time with scalability and in-turn reduced the operational cost.
Named Entity Recognition makes your machine learning models more efficient and reliable. However, you need quality training datasets for your models to work at their optimum level and achieve intended goals. All you need is a pioneer service partner who can provide you with quality datasets ready to use from unstructured data. Reach us at connect@ngenux.com for support to transform unstructured data to transformable decision-based conclusions by developing efficient and reliable ML solutions.